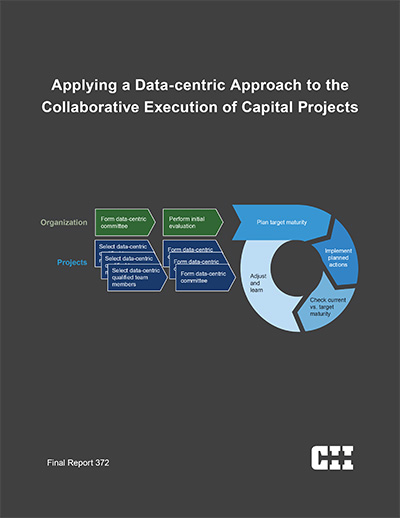
Applying a Data-centric Approach to the Collaborative Execution of Capital Projects
Data is the lifeblood of projects and organizations. Having a data-centric approach to delivering and managing facilities enables collaboration, informs decision-making, and supports advanced computational approaches such as automation and artificial intelligence. However, compared to other industries, the construction industry is one of the least digitalized industries and this shortcoming has a significant impact on its life cycle management of facilities.
To overcome this data deficit, construction organizations and project teams should expand their data-centric approaches throughout the planning, design, construction, and operations phases of a facility. According to Dell’s Digital Transformation Index, 80 percent of organizations fast-tracked at least some digital transformation programs during 2020. These higher levels of digitalization can have significant impacts on the overall success of organizations. According to a survey by McKinsey Global Institute, data-driven organizations are more likely to be profitable and have more success at acquiring and retaining customers. Another study by Franz and Messner found a significant positive relationship between digitalization practices such as BIM use adoption and the speed of delivery, perceived facility quality, and group cohesion within the project team. An essential step to the digitalization transformation of an organization is understanding and continuously improving the organization’s data-centric approaches.
CII chartered Research Team 372 (RT-372), Smart Data-centric Life Cycle Approach to Collaborative Execution of Capital Projects, to develop resources that could inform project teams and organizations about their past, current, and future levels of data-centric integration. By applying the rigorous, metric-driven approach described in this report, project teams and organizations can plan and adopt data-centric workflows and apply practices to realize the benefits of a data-centric approach. RT-372 focused on how organizations and projects teams can overcome current barriers and enable collaborative and informed approaches to embracing a data-centric integration approach to developing, executing, turning over, operating, and maintaining capital projects.
To meet its research goal, the team set out to achieve the following objectives:
- Identify the barriers to data-centric collaboration.
- Create two data-centric maturity assessment matrices – one at a project level and the other at an organizational level – along with implementation guidelines to support continuous improvement.
- Validate these data-centric maturity tools by applying them to case studies.
The team’s initial research efforts identified 22 important barriers that can limit implementing more data-driven practices. In the team’s industry survey, practicing experts singled out the following barriers as most important:
- The challenges of integrating multiple data sources
- The cost to maintain an operational model of a facility
- The organizational culture’s resistance to change
In addition, the experts named the most challenging barriers to overcome:
- The financial investments needed from small business partners or project stakeholders
- The organizational culture’s resistance to change
- The challenges of integrating multiple data sources
To help teams overcome these barriers at the organizational and project levels, RT-372 developed and tested an assessment system for measuring the maturity of the data-centric approaches of an organization or a project. The team started by reviewing existing quantitative metric systems for related technology-specific maturity tools in the industry, which required it to evaluate more than 20 such instruments. Through a content analysis of these instruments along with lessons learned to overcome important barriers, the team developed two maturity matrices that could measure the data-centric on a project or within an organization (provided as two Excel spreadsheets and included in this report as Appendices C and D).
RT-372 conducted a thorough literature review to identify barriers to the data-centric approach. This resulted in the identification of 35 barriers. Next, the team modified these barriers to better suit data-centric integration, categorizing them into the five categories show in the table (FR-372, in press).
Following its identification of the barriers to a data-centric approach, the team developed and distributed an online survey to determine the importance of each barrier and how difficult it was to overcome. Figure 1 shows the importance of each barrier on a scale from 1 to 4. Figure 2 shows the difficulty to overcome each barrier, also on a scale from 1 to 4.
{^widget|(widget_displayname)WatermarkImage|(name)WatermarkImage|(image)%7e%2fCII%2fmedia%2fPublications%2fpub_images%2fFR-372_Fig2.jpg%3fext%3d.jpg|(width)|(height)^}
Most barriers had a mean score above 2.5, indicating that the respondents found them to be important. The top four most important barriers were interoperability between software, cost to maintain an operational model of a facility, integration of multiple sources of data, and organizational cultural resistance to change. Out of these barriers, interoperability between software and integration of multiple sources of data were directly related to the data-centric approach, further highlighting the importance of a need to develop a data-centric approach in the industry.
It is important to note that the four most difficult barriers to overcome were interoperability between software, financial investment from small business partners or project stakeholders, organizational culture resistance to change, and integration of multiple sources of data. Surprisingly, organizational cultural resistance to change, interoperability between software, and integration of multiple sources of data had already been identified as important barriers. Financial investment from small business partners or project stakeholders was related to the identified importance of each barrier and the cost to maintain an operational model of a facility. Therefore, the most important barriers closely aligned with those that are difficult to overcome (FR-372, in press).
RT-372 created two data-centric maturity assessment matrices: one for the organizational level and one for the project level. Team members validated these matrices internally, reviewing and testing to ensure that they could complete the matrices in their own projects and organizations. They further tested the matrices through a series of case studies that demonstrated an alignment between the growth in organizational maturity over time and growing project-level maturity scores in the matrices over corresponding time periods. The following tables show the opening pages of both matrices (FR-372, pp. 16-19).
Click on an image below to download that spreadsheet tool.
RT-372 developed a continuous improvement process (shown in the figure below) to ensure that data-centric maturity continues to grow over time at the organizational and project levels. The framework provides a basis and process for using the matrices to perform organizational strategic planning to adopt data-centric approaches in company data requirements, collaborative processes, technical infrastructure needs, personnel and training needs, as well as identifying use cases for the data. The process for implementing the data-centric approach at the organizational level aligns with its adoption in the organization’s capital projects. In this way, the projects’ adoption of data-centric advances the development and handover of data that advance the organization’s implementation (FR-372, pp. 20).